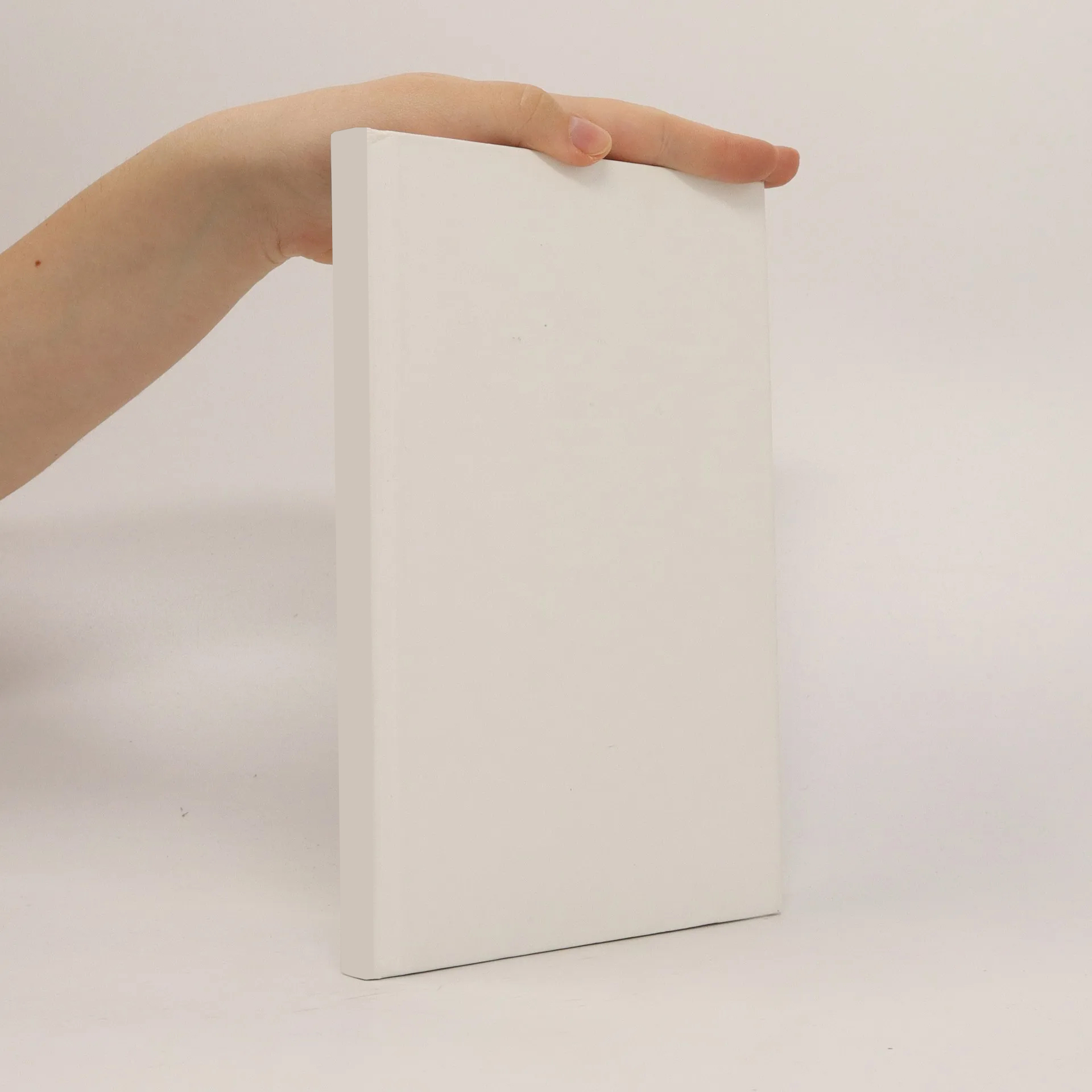
Interactive and life-long learning for identification and categorization tasks
Authors
More about the book
This thesis focuses on life-long and interactive learning for recognition tasks. To achieve these targets the separation into a short-term memory (STM) and a longterm memory (LTM) is proposed. For the incremental build up of the STM a similarity-based one-shot learning method was developed. Furthermore two consolidation algorithms were proposed enabling the incremental learning of LTM representations. Based on the Learning Vector Quantization (LVQ) network architecture an error-based node insertion rule and a node dependent learning rate are proposed to enable life-long learning. For learning of categories additionally a forward-feature selection method was introduced to separate co-occurring categories. In experiments the performance of these learning methods could be shown for difficult visual recognition problems.