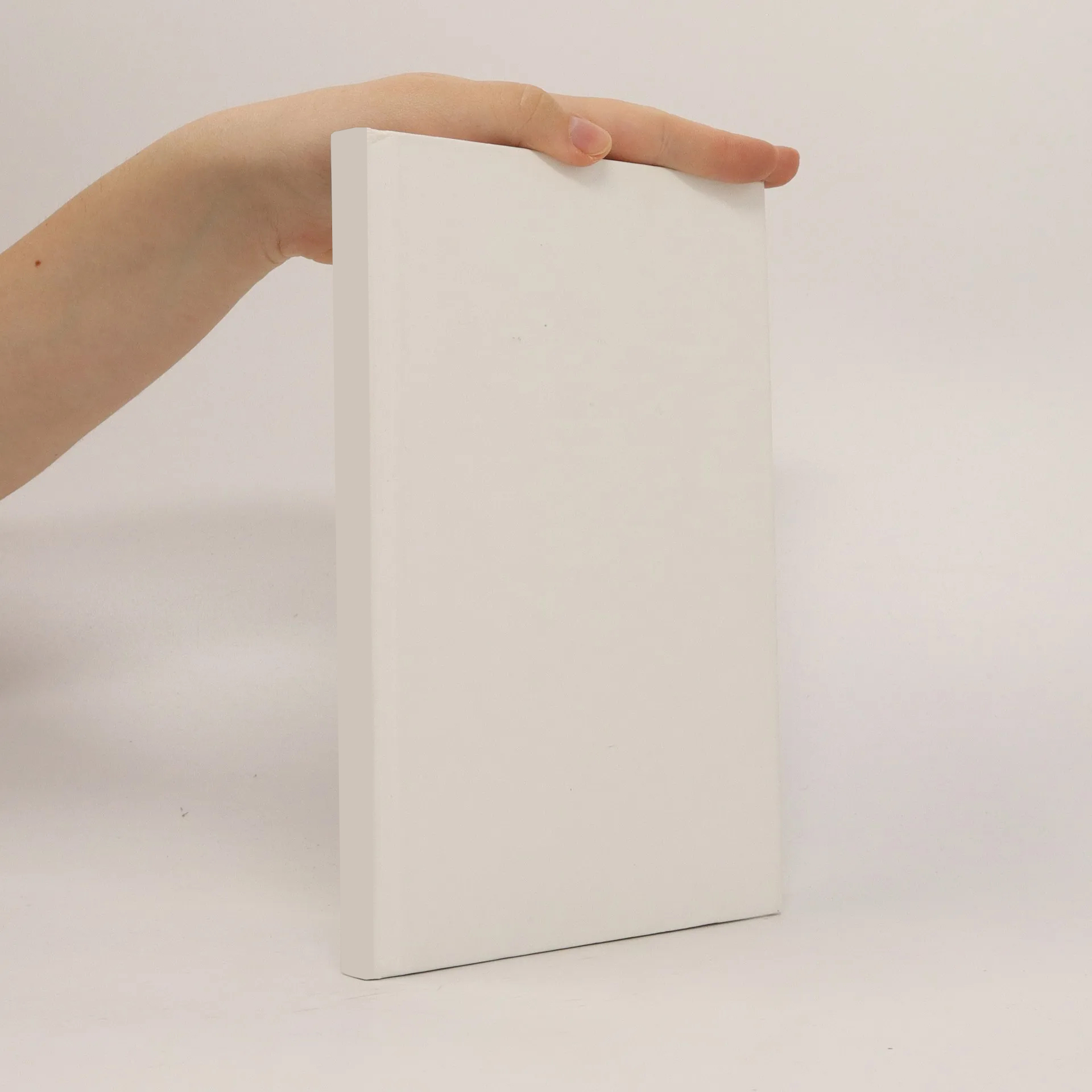
Data-driven adaptive robust control based upon unfalsified control
Authors
More about the book
The focus of this thesis is on the development of an adaptive control scheme which is applicable to a wide class of systems and provides a close to optimum performance. To achieve this goal, a data-driven adaptive control approach called unfalsified control is used. The key feature of unfalsified control is the fact that very little knowledge about the plant is required a priori, hence it can be easily applied to a large class of systems. The basic idea of unfalsified control is to switch between finitely many, predefined controllers in a set based upon measured data. To improve the set of candidate controllers, the original idea of unfalsified control has recently been extended to include an optimization of the elements in the set of controllers. In this thesis, the main findings in the literature on unfalsified control are summarized and a framework for adaptive unfalsified control is presented and further developed. A new transfer approach to facilitate the adaptation of the controller parameters is presented. A performance monitoring mechanism is developed to trigger the optimization efficiently. It is shown why robustness is required in unfalsified control framework, and a novel approach to include robustness into the unfalsified control framework is developed. How the presented adaptive control scheme works is exemplified by three realistic and challenging case studies from chemical engineering. The examples show how the proposed method improves the performance of the closed-loop by adapting the active controller parameters during plant operation. The results are then compared with the performance of fixed non-adaptive controllers in case of disturbance rejection and set-point tracking scenarios.