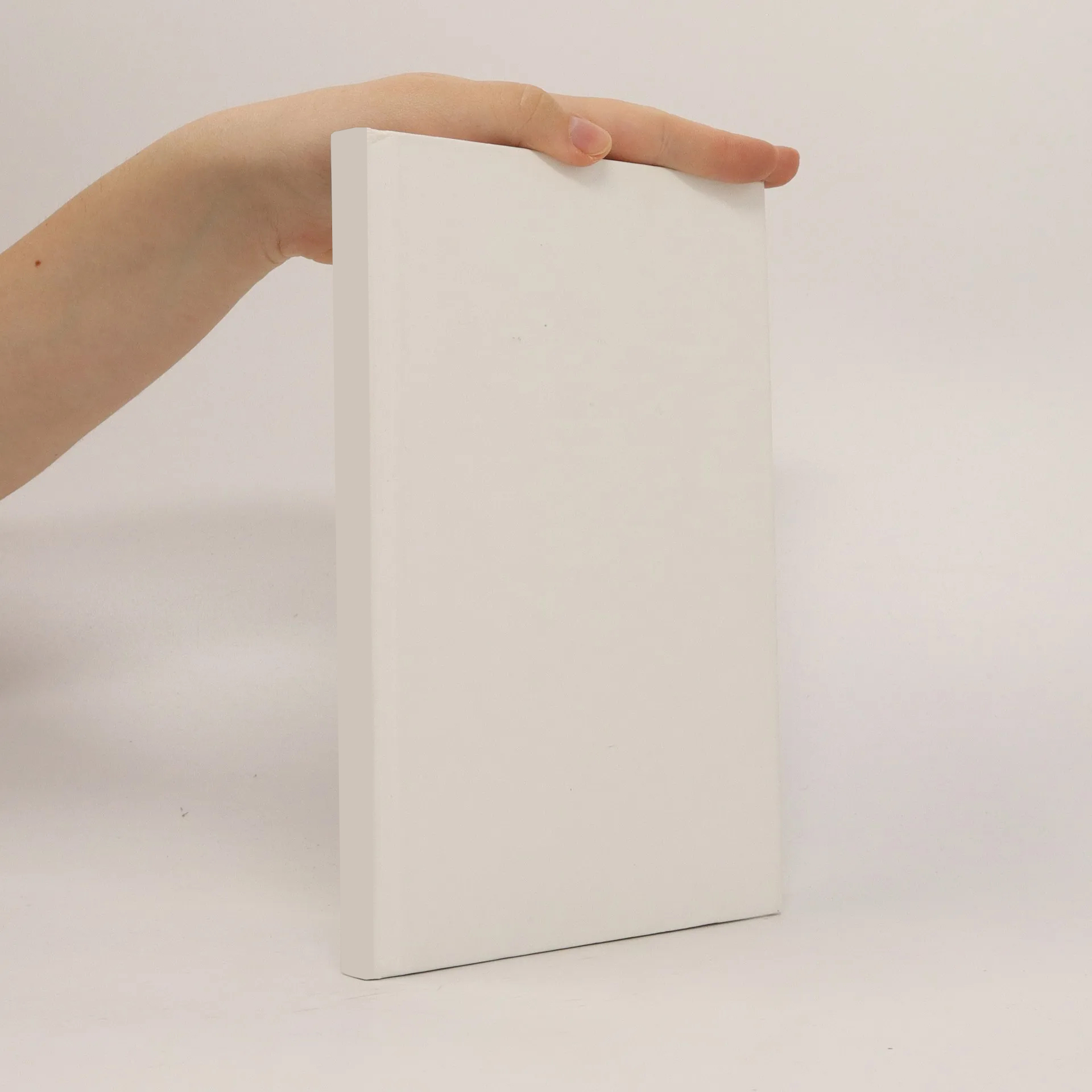
Predictive control under uncertainty
Authors
Parameters
Categories
More about the book
This thesis addresses constrained control of systems under stochastic disturbances and model uncertainty. In particular, stochastic and adaptive model predictive control (MPC) algorithms that solve such problems are presented and studied. Given a stochastic disturbance model, the focus is put on chance constraints, tractable approximations, and their implication on feasibility of the online optimization in a receding horizon framework. For systems with model uncertainty, online parameter identification to reduce conservatism and improve closed-loop performance is addressed. More specifically, building upon the analysis of conceptual aspects, we develop computational approaches for linear systems with additive and multiplicative disturbances. The analysis of a non-conservative, computationally tractable relaxation of chance constraints leads to an important separation of sufficient conditions for feasibility and stability. The latter is of particular interest for rigorously applying approximations, such as finite sample approximations, to solve the online stochastic optimal control problem. We discuss the differences between online and offline sampling approximations and, for systems that are linear in the state and input variables, provide explicit bounds on the sample complexity to guarantee satisfaction of chance constraints with a user chosen confidence. The proposed algorithms provide rigorous guarantees for relevant properties like feasibility of the online optimization, constraint satisfaction, and convergence of the closed-loop system.
Book purchase
Predictive control under uncertainty, Matthias Lorenzen
- Language
- Released
- 2019
Payment methods
- Title
- Predictive control under uncertainty
- Language
- English
- Authors
- Matthias Lorenzen
- Publisher
- Logos
- Released
- 2019
- ISBN10
- 3832549803
- ISBN13
- 9783832549800
- Category
- University and college textbooks
- Description
- This thesis addresses constrained control of systems under stochastic disturbances and model uncertainty. In particular, stochastic and adaptive model predictive control (MPC) algorithms that solve such problems are presented and studied. Given a stochastic disturbance model, the focus is put on chance constraints, tractable approximations, and their implication on feasibility of the online optimization in a receding horizon framework. For systems with model uncertainty, online parameter identification to reduce conservatism and improve closed-loop performance is addressed. More specifically, building upon the analysis of conceptual aspects, we develop computational approaches for linear systems with additive and multiplicative disturbances. The analysis of a non-conservative, computationally tractable relaxation of chance constraints leads to an important separation of sufficient conditions for feasibility and stability. The latter is of particular interest for rigorously applying approximations, such as finite sample approximations, to solve the online stochastic optimal control problem. We discuss the differences between online and offline sampling approximations and, for systems that are linear in the state and input variables, provide explicit bounds on the sample complexity to guarantee satisfaction of chance constraints with a user chosen confidence. The proposed algorithms provide rigorous guarantees for relevant properties like feasibility of the online optimization, constraint satisfaction, and convergence of the closed-loop system.