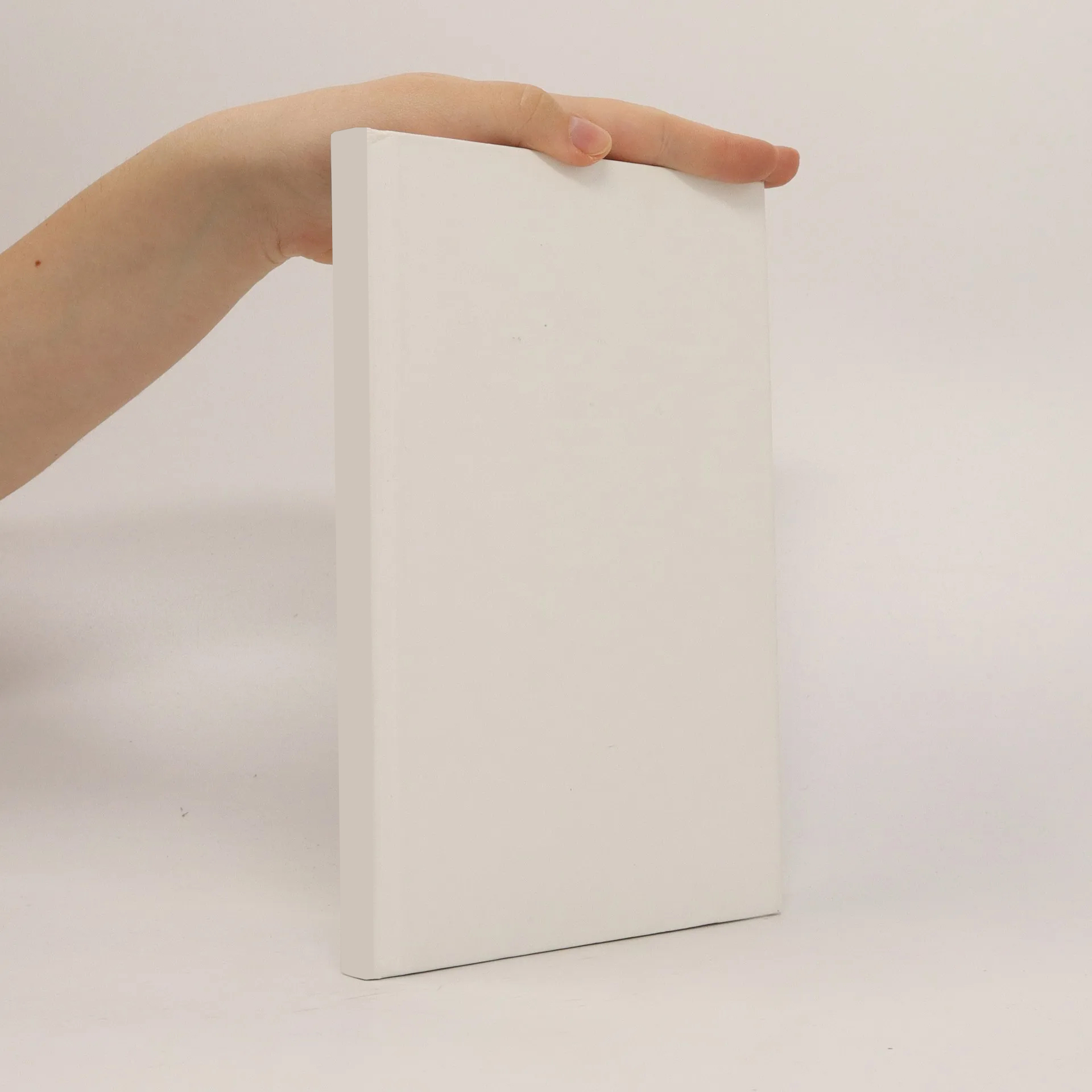
The matrix measure framework for projection-based model order reduction
Authors
More about the book
Nowadays, scientists and engineers frequently require well-detailed numerical models of complex physical systems to perform simulations, optimization, design or control. However, these accurate models are often defined by a large number of equations, which can be unmanageable with the current data processors due to the problems caused by the curse of dimensionality. This challenge can be overcome by Model Order Reduction (MOR), which consists of reducing the model complexity, i. e. the number of involved variables, while preserving the overall input-output behaviour of the model. This thesis presents a new framework for guaranteeing stability and ensuring performance in projection-based MOR. The framework is based on the mathematical concept of matrix measure, can be applied to several MOR algorithms and is capable of dealing with large-scale systems. Moreover, this approach offers new solutions to a set of open problems in this research field; namely, stability preservation in MOR of continuous, discrete, and parametric systems, and the definition of system norms and indicators, which are required to evaluate the quality of approximation. As particularly interesting aspects, this general framework links several other contributions in the area, and moreover, can be applied without any additional numerical effort to a large set of industry-relevant MOR problems. Furthermore, a number of results in the literature of linear algebra regarding the matrix measure, norms and matrix balancing are now linked to the area of MOR of dynamical systems, offering new ideas and solutions.