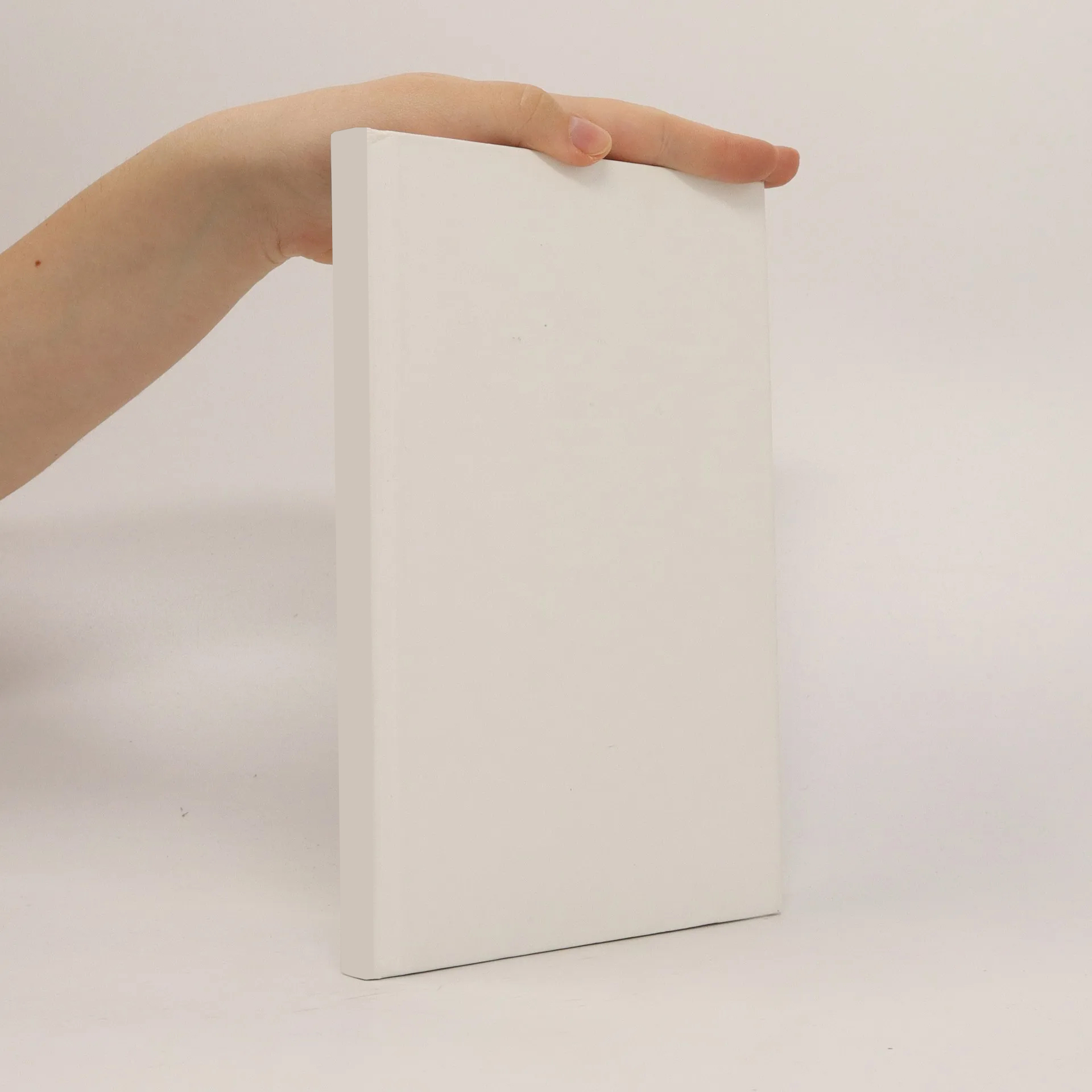
More about the book
This ready reference discusses different methods for statistically analyzing and validating data created with high-throughput methods. As opposed to other titles, this book focusses on systems approaches, meaning that no single gene or protein forms the basis of the analysis but rather a more or less complex biological network. From a methodological point of view, the well balanced contributions describe a variety of modern supervised and unsupervised statistical methods applied to various large-scale datasets from genomics and genetics experiments. Furthermore, since the availability of sufficient computer power in recent years has shifted attention from parametric to nonparametric methods, the methods presented here make use of such computer-intensive approaches as Bootstrap, Markov Chain Monte Carlo or general resampling methods. Finally, due to the large amount of information available in public databases, a chapter on Bayesian methods is included, which also provides a systematic means to integrate this information. A welcome guide for mathematicians and the medical and basic research communities.
Book purchase
Statistical diagnostics for cancer, Frank Emmert
- Language
- Released
- 2013
- product-detail.submit-box.info.binding
- (Hardcover)
Payment methods
No one has rated yet.