The book is currently out of stock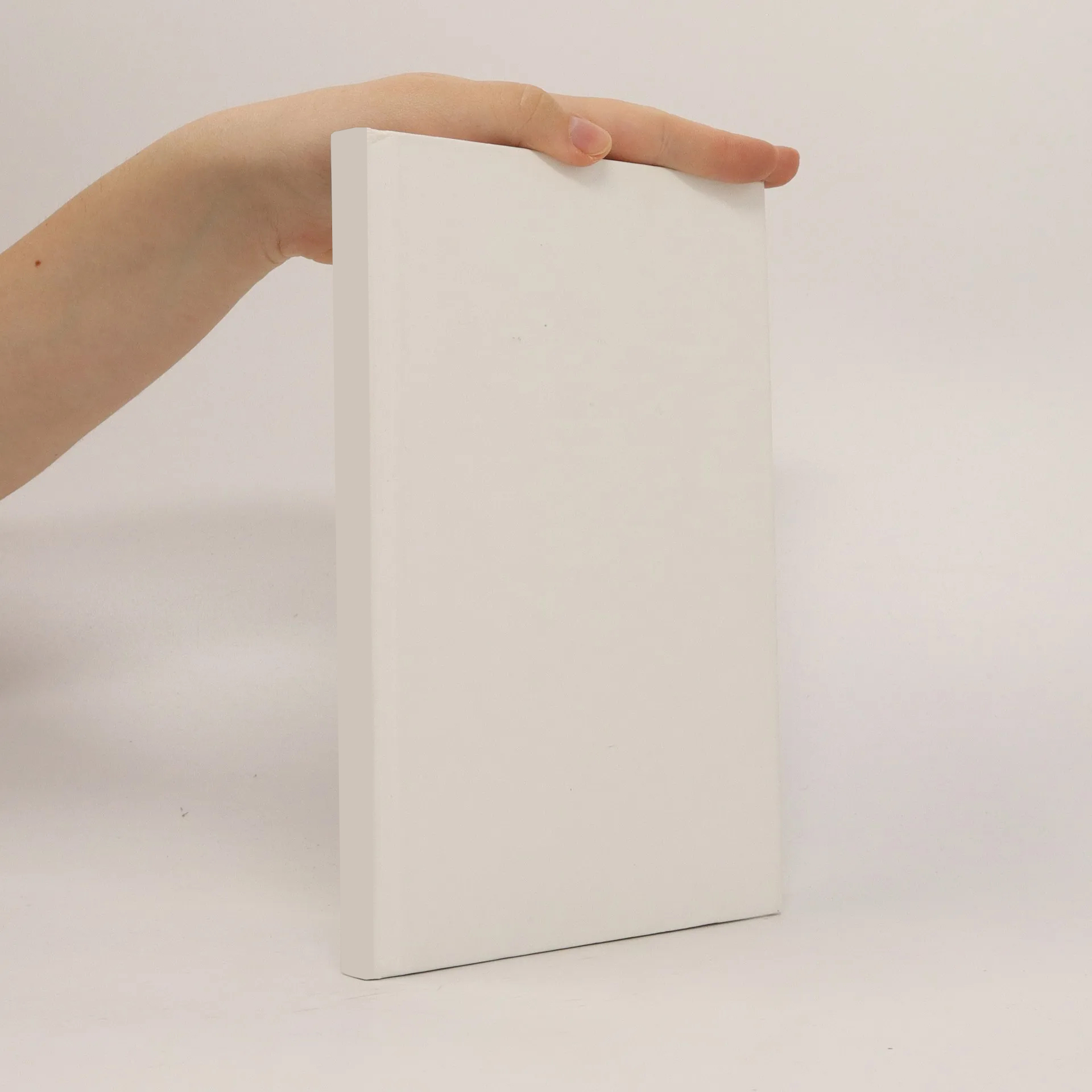
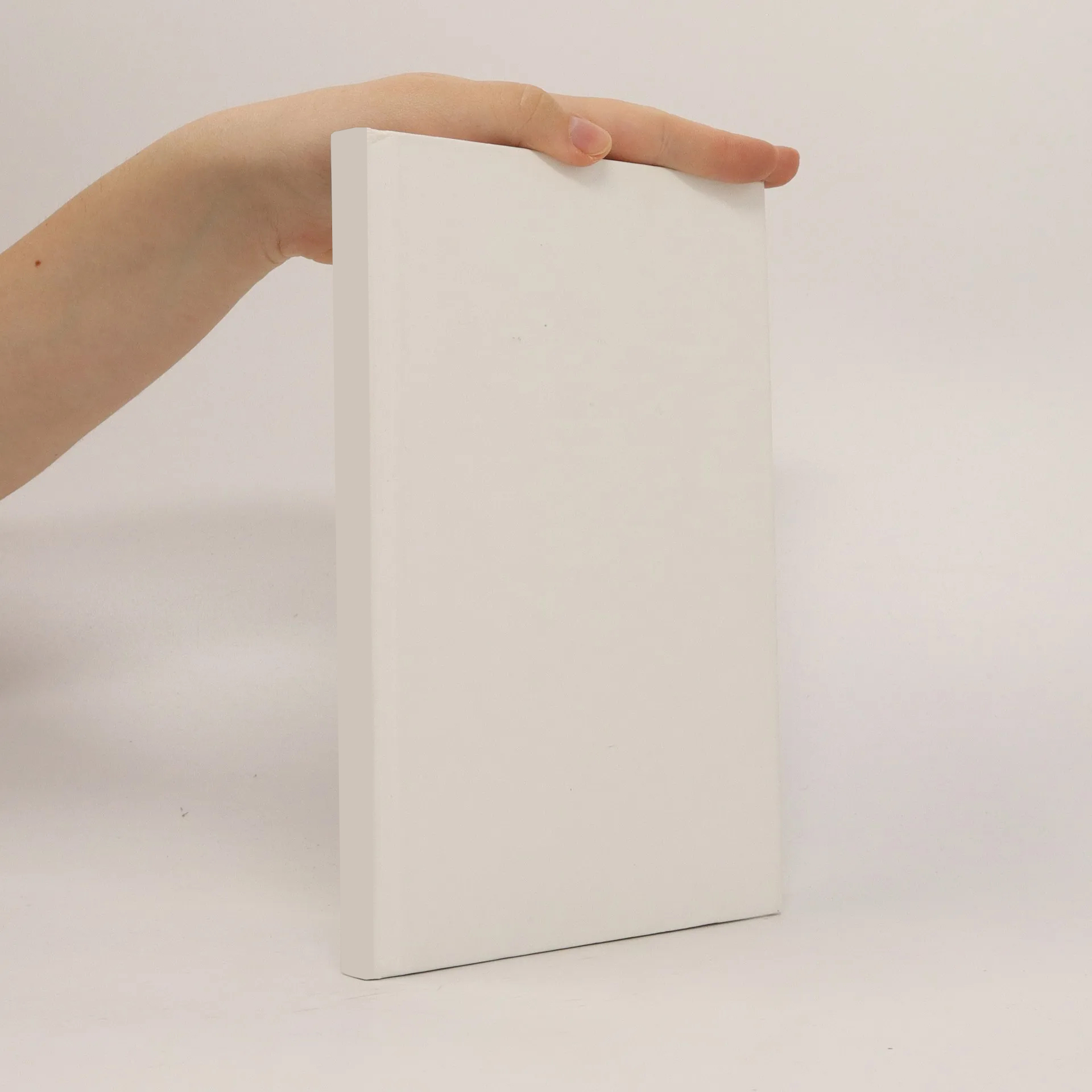
More about the book
Focusing on ensemble learning, this work highlights the effectiveness of combining diverse neural network classifiers to achieve improved accuracy over single models. It examines how randomizing neural network parameters can create diverse ensembles, enhancing generalization. By employing a sampling strategy akin to Random Forests, the study aims to foster disagreement among network members. Experimental findings reveal that while inducing diversity in ensembles can be beneficial, it does not always guarantee accuracy improvements, making this research valuable for enthusiasts of ensemble methods and neural networks.
Book purchase
Towards Optimally Diverse Randomized Ensembles of Neural Networks, Anna Martin
- Language
- Released
- 2017
- product-detail.submit-box.info.binding
- (Paperback)
We’ll email you as soon as we track it down.
Payment methods
No one has rated yet.